Book Downloads Hub Reads Ebooks Online eBook Librarys Digital Books Store Download Book Pdfs Bookworm Downloads Book Library Help Epub Book Collection Pdf Book Vault Read and Download Books Open Source Book Library Best Book Downloads G L Creasy Saxon Andrew John Stuhl Dore Gold Mark S Leclair Curt Sampson Shana Goldin Perschbacher Philip Hasheider
Do you want to contribute by writing guest posts on this blog?
Please contact us and send us a resume of previous articles that you have written.
Neural Networks In Finance - Revolutionizing the Way We Trade

Throughout history, financial markets have always been a complex set of intertwined variables that are influenced by countless factors. Investors and traders constantly seek an edge by analyzing huge amounts of data and trying to predict market movements. Traditionally, this task required human intuition, experience, and a deep understanding of market dynamics. However, the advent of neural networks has transformed the financial landscape, introducing a new era of data-driven decision-making.
Neural networks, inspired by the human brain, are a powerful tool that can learn complex patterns and relationships from vast amounts of data. When applied to finance, they can uncover hidden insights, predict market trends, and optimize investment strategies. Let's explore how neural networks revolutionize the way we trade.
The Power of Neural Networks
Neural networks have gained popularity in finance due to their ability to process information much faster and more accurately than traditional methods. These networks consist of interconnected artificial neurons or nodes that receive inputs, process them through weighted connections, and generate outputs. By using historical financial data as training sets, neural networks can recognize patterns, extract meaningful features, and make predictions.
4.2 out of 5
Language | : | English |
File size | : | 11677 KB |
Text-to-Speech | : | Enabled |
Screen Reader | : | Supported |
Enhanced typesetting | : | Enabled |
Print length | : | 352 pages |
One remarkable advantage of neural networks is their ability to handle non-linear relationships. Unlike linear regression or time series analysis, neural networks can capture complex and non-linear patterns that exist in financial markets. This opens up possibilities for more accurate and robust predictions, which can be crucial for traders seeking profitable opportunities.
The flexibility of neural networks also allows for customization. Depending on the complexity and specific requirements of a financial problem, the architecture and structure of the neural network can be tuned. This adaptability makes neural networks a versatile tool that can be applied across different market conditions, trading strategies, and asset classes.
Predictive Modeling and Trading Strategies
One of the most powerful applications of neural networks in finance is predictive modeling. By analyzing vast historical data, neural networks can identify significant patterns and generate forecasts on various financial parameters such as stock prices, interest rates, exchange rates, and commodities. These predictions can help investors make informed decisions and improve risk management.
Neural networks can also assist in developing trading strategies by identifying signals, trends, and anomalies in financial markets. They can automatically detect patterns associated with potential profitable opportunities and generate buy/sell signals accordingly. With their ability to adapt to changing market conditions, neural networks can continuously learn and evolve, ensuring trading strategies remain effective.
Furthermore, neural networks can aid in creating more accurate risk models. By incorporating a vast array of financial data, these models can estimate the probability of a certain event occurring, such as a market crash or a company bankruptcy. Financial institutions can utilize these models to enhance their risk management processes, making them better equipped to handle unexpected market fluctuations.
Challenges and Future Outlook
While neural networks offer numerous advantages in the finance industry, their implementation is not without challenges. One common limitation is the "black box" nature of neural networks. Unlike traditional statistical models, neural networks do not provide explicit explanations for their decisions, which can make it difficult for humans to interpret their predictions and understand the underlying rationale.
Another challenge is the data quality and quantity required for training neural networks. Financial data can be noisy, non-stationary, and subject to various biases. Additionally, acquiring labeled data for supervised learning tasks can be resource-intensive and time-consuming. Overcoming these challenges requires robust data collection and preprocessing techniques to ensure the accuracy and reliability of neural network predictions.
Looking forward, the future of neural networks in finance appears promising. As computational power continues to increase, neural networks will become even more sophisticated, capable of handling massive amounts of data and tackling more complex financial problems. With advancements in deep learning algorithms and techniques, we can expect improved performance, increased interpretability, and enhanced risk management capabilities.
Neural networks have undoubtedly revolutionized the way we approach finance. From predictive modeling and trading strategies to risk management, these powerful tools offer insights and capabilities that were once unimaginable. As technology advances and our understanding of neural networks deepens, we can expect them to play an increasingly significant role in shaping the future of finance, enabling better decision-making and ultimately, increasing profitability.
4.2 out of 5
Language | : | English |
File size | : | 11677 KB |
Text-to-Speech | : | Enabled |
Screen Reader | : | Supported |
Enhanced typesetting | : | Enabled |
Print length | : | 352 pages |
This book explores the intuitive appeal of neural networks and the genetic algorithm in finance. It demonstrates how neural networks used in combination with evolutionary computation outperform classical econometric methods for accuracy in forecasting, classification and dimensionality reduction.
McNelis utilizes a variety of examples, from forecasting automobile production and corporate bond spread, to inflation and deflation processes in Hong Kong and Japan, to credit card default in Germany to bank failures in Texas, to cap-floor volatilities in New York and Hong Kong.
* Offers a balanced, critical review of the neural network methods and genetic algorithms used in finance
* Includes numerous examples and applications
* Numerical illustrations use MATLAB code and the book is accompanied by a website
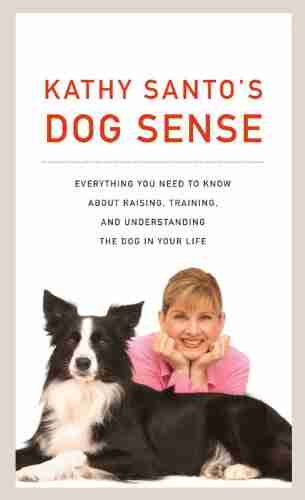

Kathy Santo Dog Sense Kathy Santo - Unlocking the secrets...
Are you a dog lover who...
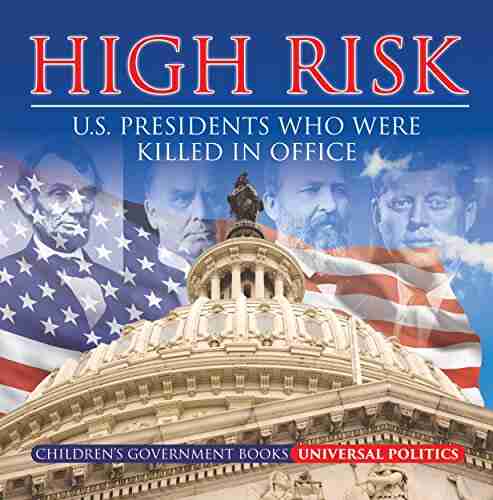

10 Presidents Who Were Killed In Office - Shocking Truth...
Throughout history, the role of a president...
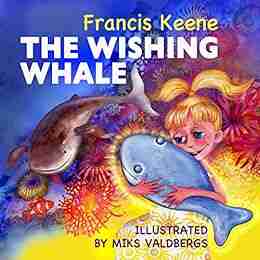

Unveiling a World of Magic: Beautifully Illustrated...
Bedtime stories have always held a...
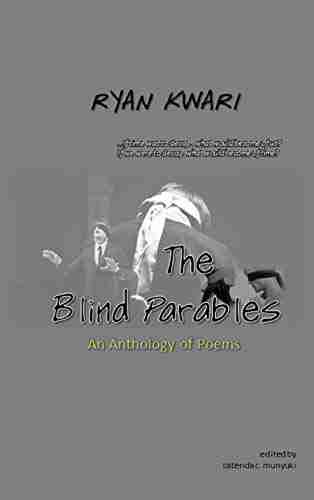

The Blind Parables: An Anthology Of Poems
For centuries, poetry has...
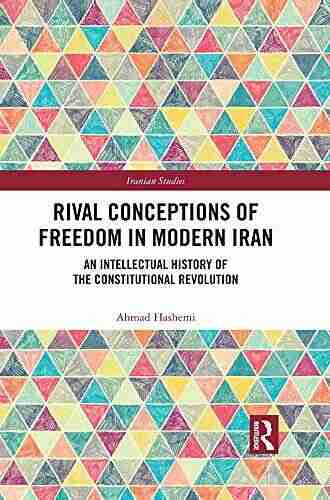

Rival Conceptions Of Freedom In Modern Iran
The Struggle for Freedom in...
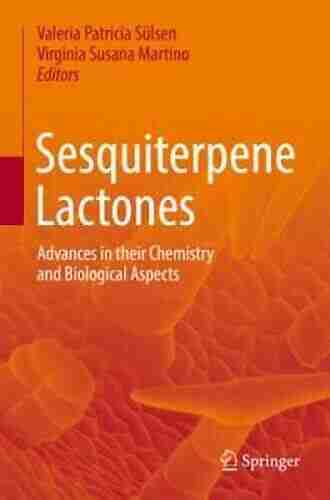

Advances In Their Chemistry And Biological Aspects
In recent years,...
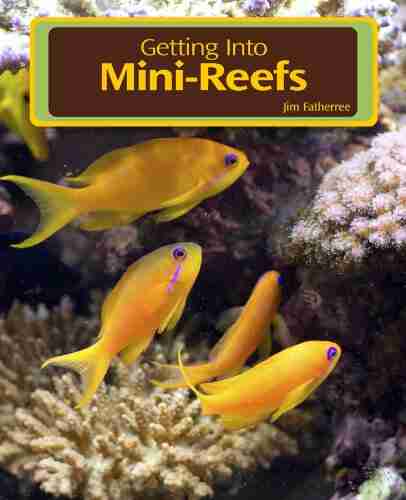

Getting Into Mini Reefs For The Marine Aquarium
Are you interested in enhancing the...
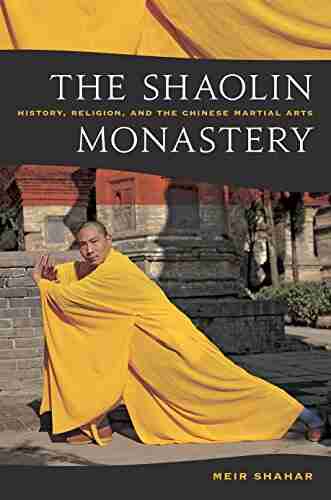

Exploring the Intriguing Connection Between History,...
When one thinks of Chinese martial...
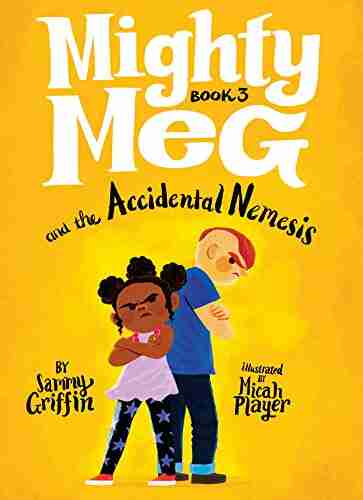

Mighty Meg And The Accidental Nemesis: Unleashing the...
In the world of superheroes, there are many...
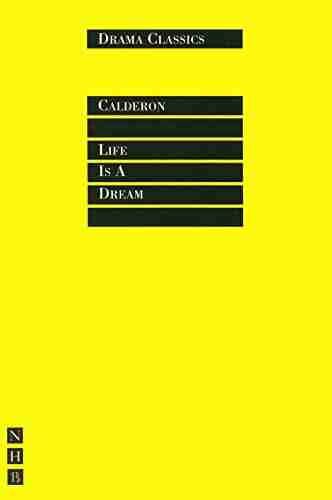

A Journey through the World of Nhb Drama Classics: Full...
Welcome to a fascinating exploration of Nhb...
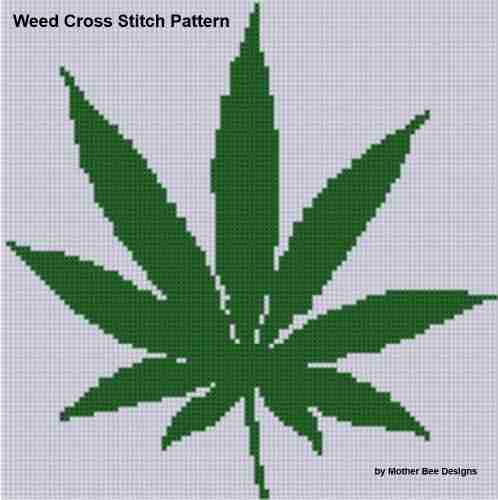

Weed Cross Stitch Pattern Rachel Worth - The Perfect...
Are you a stoner who loves a little...
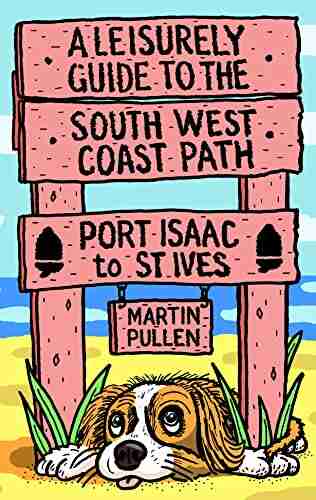

Discover the Breathtaking Beauty of the South West Coast...
Are you ready for an...
Light bulbAdvertise smarter! Our strategic ad space ensures maximum exposure. Reserve your spot today!
- Dallas TurnerFollow ·18.9k
- Eddie PowellFollow ·17.2k
- Matthew WardFollow ·5.8k
- Ernest ClineFollow ·5.4k
- Fernando BellFollow ·9.1k
- Caleb LongFollow ·6.6k
- Dominic SimmonsFollow ·15.3k
- Felix HayesFollow ·12.2k