Book Downloads Hub Reads Ebooks Online eBook Librarys Digital Books Store Download Book Pdfs Bookworm Downloads Book Library Help Epub Book Collection Pdf Book Vault Read and Download Books Open Source Book Library Best Book Downloads Susan Fox Rogers John Emsley Lisa Kemmerer Fanny Burney Nicki Grace Emma Couples Dr Hoe Bing Lo Dean Moyar
Do you want to contribute by writing guest posts on this blog?
Please contact us and send us a resume of previous articles that you have written.
Unlocking the Secrets: A Definitive Guide to Estimation and Inference for Nonlinear Dynamic Models

Are you ready to dive into the fascinating world of nonlinear dynamic models? We have the ultimate guide for you! In this comprehensive article, we will provide you with a step-by-step approach to estimating and inferring the parameters of these complex models. Get ready to unravel the mysteries and gain a deeper understanding of nonlinear systems.
to Nonlinear Dynamic Models
Nonlinear dynamic models are mathematical representations used to describe the behavior of complex systems that cannot be easily captured by linear models. These models find applications in various fields, including economics, physics, biology, and engineering, where the relationships among variables are intricate and nonlinear.
Understanding and analyzing nonlinear dynamic models require advanced statistical techniques. This guide aims to equip you with the knowledge and tools needed to estimate the parameters accurately and make meaningful inferences from these models.
5 out of 5
Language | : | English |
File size | : | 12624 KB |
Screen Reader | : | Supported |
Print length | : | 378 pages |
Item Weight | : | 3.52 ounces |
Paperback | : | 45 pages |
Dimensions | : | 7 x 0.11 x 10 inches |
Estimation Techniques
Estimating the parameters of nonlinear dynamic models is a challenging task due to the complexity and nonlinearity of the relationships involved. However, several methods have been developed to tackle this problem effectively. Let's explore some of the most widely used estimation techniques:
1. Maximum Likelihood Estimation (MLE)
MLE is a popular technique used to estimate the parameters in statistical models, including nonlinear dynamic models. It involves finding the set of parameters that maximize the likelihood of the observed data given the model. MLE provides a powerful framework for parameter estimation and forms the basis of many other estimation methods.
2. Sequential Monte Carlo (SMC) Methods
SMC methods, also known as particle filters, are a class of algorithms used to estimate the states and parameters of nonlinear dynamic models. These methods rely on a set of particles that represent possible states of the system. By iteratively updating the weights of these particles based on observed data, SMC methods provide an approximation of the posterior distribution of the parameters.
3. Bayesian Inference
Bayesian inference is a powerful framework for estimating parameters in nonlinear dynamic models. It involves assigning prior distributions to the parameters and updating them based on observed data using Bayes' theorem. Bayesian inference provides a way to quantify uncertainty and make predictions in a principled manner.
Inference Techniques
Once the parameters have been estimated, it is crucial to perform inference to draw meaningful s from the model. Let's explore some popular inference techniques used in nonlinear dynamic models:
1. Hypothesis Testing
Hypothesis testing allows us to test the significance of parameters and model assumptions in nonlinear dynamic models. By formulating null and alternative hypotheses, we can evaluate the evidence against or in favor of a specific claim. Various statistical tests, such as t-tests and likelihood ratio tests, can be employed to perform hypothesis testing.
2. Confidence Intervals
Confidence intervals provide a range of plausible values for the model parameters. They quantify the uncertainty associated with parameter estimation and can help assess the precision of the estimates. Confidence intervals are often computed based on the asymptotic distribution of the estimators or using bootstrap methods.
3. Model Comparison
Model comparison is crucial when dealing with nonlinear dynamic models with different specifications or assumptions. Techniques like information criteria (e.g., AIC and BIC) and likelihood ratio tests can be employed to compare the relative performance of competing models. Model comparison helps researchers select the most appropriate model for their data.
Nonlinear dynamic models provide a powerful framework for understanding the complexity and dynamics of various real-world systems. Estimating the parameters accurately and making meaningful inferences from these models require advanced statistical techniques. In this guide, we covered various estimation and inference techniques, including maximum likelihood estimation, sequential Monte Carlo methods, and Bayesian inference.
Remember, mastering the estimation and inference of nonlinear dynamic models is a journey that requires practice and continuous learning. By familiarizing yourself with these techniques and applying them to your own research, you can unlock the secrets hidden within nonlinear systems and make significant contributions to your field.
So, are you ready to embark on this exciting journey? Let's explore the wonderful world of nonlinear dynamic models together!
5 out of 5
Language | : | English |
File size | : | 12624 KB |
Screen Reader | : | Supported |
Print length | : | 378 pages |
Item Weight | : | 3.52 ounces |
Paperback | : | 45 pages |
Dimensions | : | 7 x 0.11 x 10 inches |
This graduate textbook covers the theory and practice of econometrics and data science in complex nonlinear dynamic settings. The reader is guided through a range of modern topics in statistics and data science for nonlinear models, including, (i) estimation theory for analytically intractable estimators, (ii) econometric analysis of misspecified models, (iii) optimal model and estimator selection, and (iv) structural and causal modeling. The methods and techniques covered in this textbook are applied extensively to nonlinear dynamic models, time-series filtering techniques, and dynamic non-parametric methods.
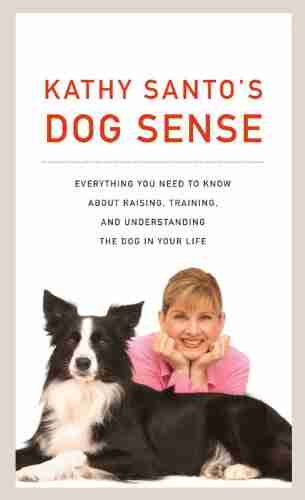

Kathy Santo Dog Sense Kathy Santo - Unlocking the secrets...
Are you a dog lover who...
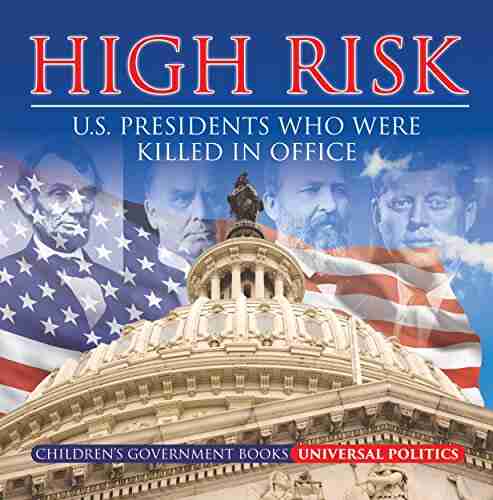

10 Presidents Who Were Killed In Office - Shocking Truth...
Throughout history, the role of a president...
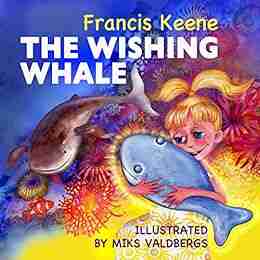

Unveiling a World of Magic: Beautifully Illustrated...
Bedtime stories have always held a...
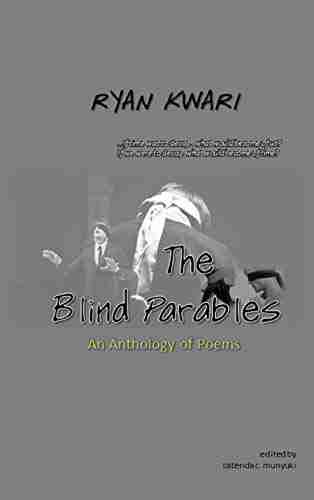

The Blind Parables: An Anthology Of Poems
For centuries, poetry has...
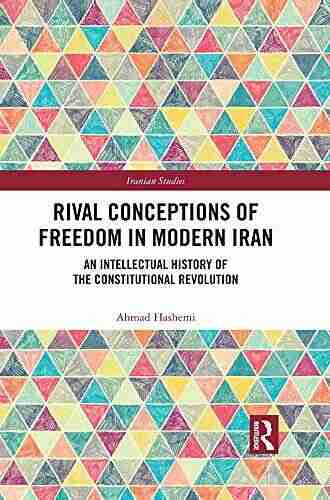

Rival Conceptions Of Freedom In Modern Iran
The Struggle for Freedom in...
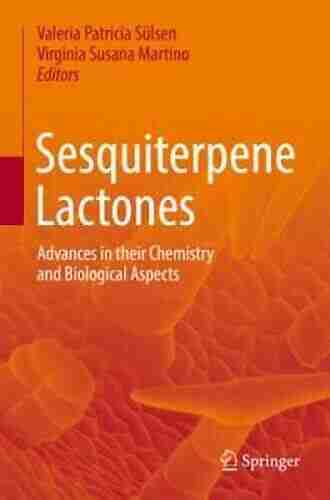

Advances In Their Chemistry And Biological Aspects
In recent years,...
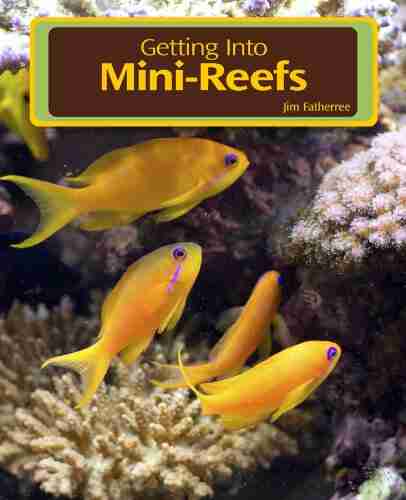

Getting Into Mini Reefs For The Marine Aquarium
Are you interested in enhancing the...
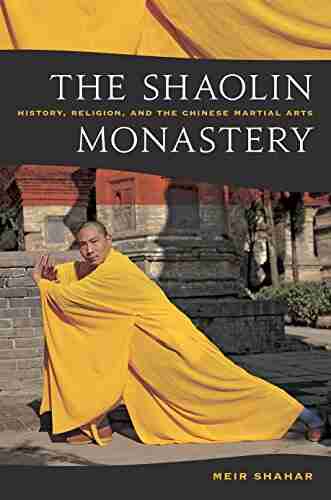

Exploring the Intriguing Connection Between History,...
When one thinks of Chinese martial...
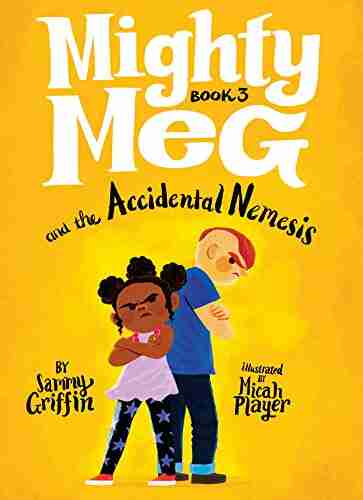

Mighty Meg And The Accidental Nemesis: Unleashing the...
In the world of superheroes, there are many...
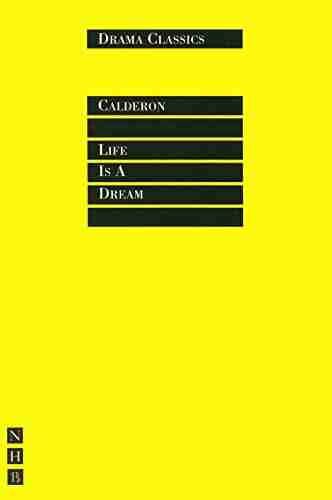

A Journey through the World of Nhb Drama Classics: Full...
Welcome to a fascinating exploration of Nhb...
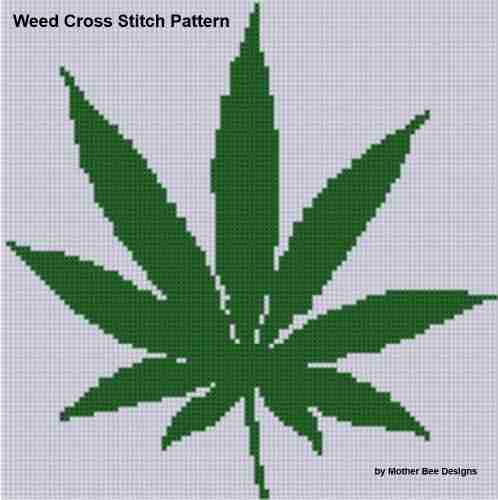

Weed Cross Stitch Pattern Rachel Worth - The Perfect...
Are you a stoner who loves a little...
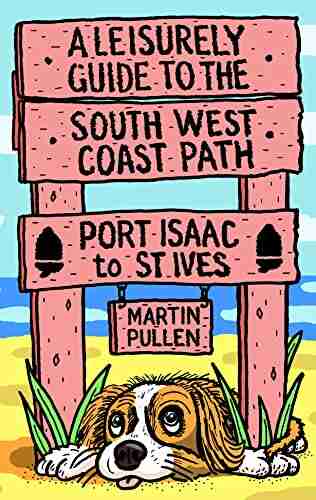

Discover the Breathtaking Beauty of the South West Coast...
Are you ready for an...
Light bulbAdvertise smarter! Our strategic ad space ensures maximum exposure. Reserve your spot today!
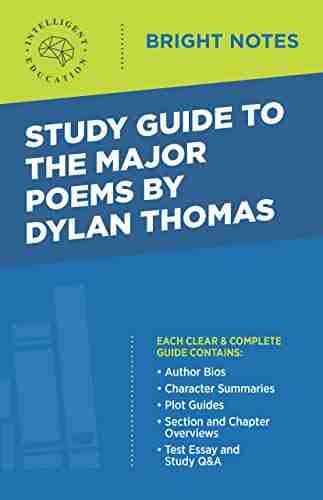

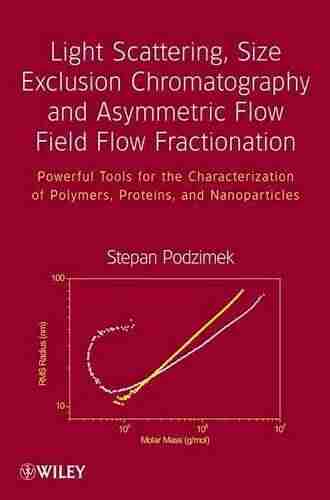

- Shaun NelsonFollow ·2.5k
- Octavio PazFollow ·10.7k
- Cade SimmonsFollow ·19k
- Jared NelsonFollow ·5.5k
- Mike HayesFollow ·14.4k
- Edward ReedFollow ·10k
- Scott ParkerFollow ·14.5k
- Wesley ReedFollow ·14.9k