Book Downloads Hub Reads Ebooks Online eBook Librarys Digital Books Store Download Book Pdfs Bookworm Downloads Book Library Help Epub Book Collection Pdf Book Vault Read and Download Books Open Source Book Library Best Book Downloads Francis Keene Carl Wennerlind Robin Koontz Paul Henderson Erica N Walker Claire Foster Gilbert Daniel E Kelly Team 2030
Do you want to contribute by writing guest posts on this blog?
Please contact us and send us a resume of previous articles that you have written.
Unveiling the Secrets of Advanced Deep Learning with Tensorflow and Keras

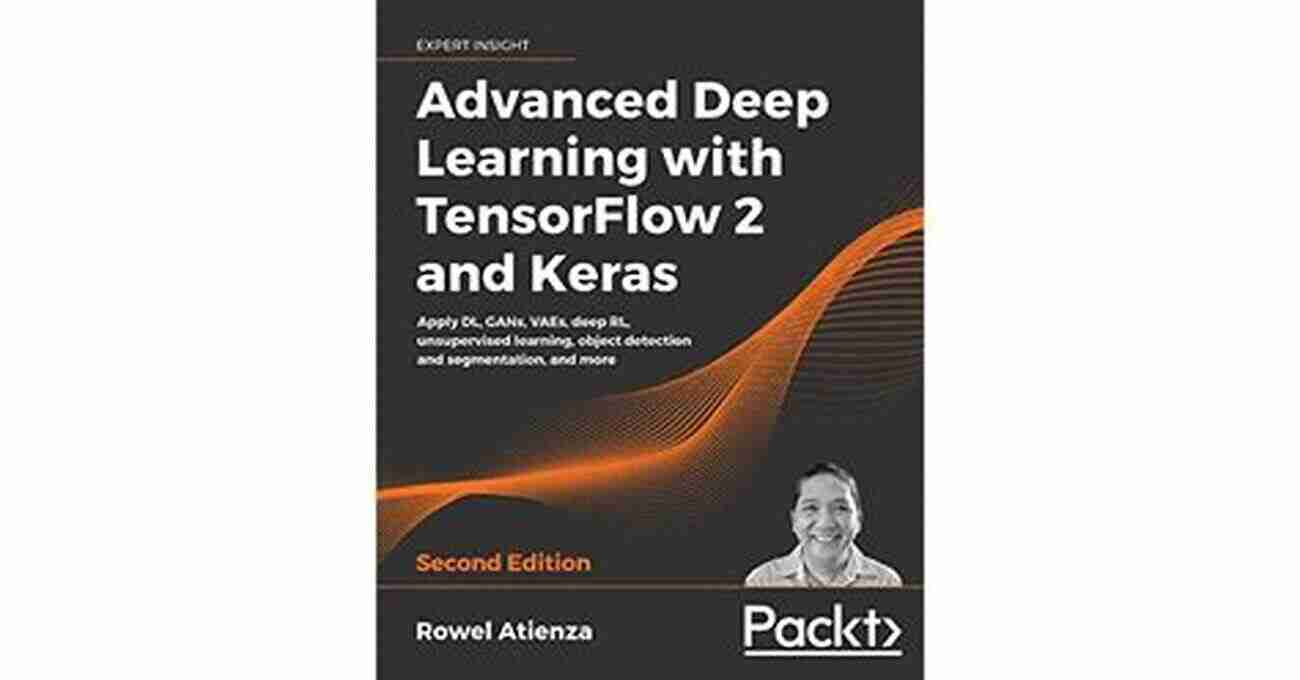
Deep learning has emerged as one of the most prominent fields in artificial intelligence (AI) and machine learning. Its ability to analyze large amounts of complex data and make intelligent decisions has revolutionized various industries, including healthcare, finance, and technology. Among the wide range of deep learning frameworks available, Tensorflow and Keras stand out for their flexibility, scalability, and ease of use.
Understanding Deep Learning
In simple terms, deep learning is a subset of machine learning that focuses on training artificial neural networks with multiple layers to mimic the human brain's behavior. It involves the use of neural networks with interconnected nodes, or artificial neurons, that can learn from vast amounts of data. By processing this data, deep learning models can identify patterns, make predictions, and perform various complex tasks.
Tensorflow: Powering Advanced Deep Learning
Tensorflow, developed by Google, has become the go-to framework for deep learning. With its robust ecosystem, it enables developers to build and deploy advanced models across different platforms. Tensorflow provides a vast array of pre-built operations, called ops, that facilitate building complex neural networks. These ops are designed to efficiently handle large-scale datasets, making Tensorflow an ideal choice for advanced deep learning projects.
4.4 out of 5
Language | : | English |
File size | : | 28714 KB |
Text-to-Speech | : | Enabled |
Screen Reader | : | Supported |
Enhanced typesetting | : | Enabled |
Print length | : | 512 pages |
Keras: Simplifying Deep Learning
While Tensorflow offers tremendous power and flexibility, Keras complements it by providing a simplified interface for building deep learning models. Keras acts as a user-friendly front-end API for Tensorflow, allowing developers to quickly prototype and experiment with different architectures. Keras excels in enabling rapid development, making it a favorite among beginners and experts alike.
Applying Advanced Techniques
Deep learning with Tensorflow and Keras opens doors to a wide range of advanced techniques that can enhance model performance and contribute to solving complex problems. Some of these techniques include:
- Convolutional Neural Networks (CNNs) for image recognition and computer vision tasks
- Recurrent Neural Networks (RNNs) for sequence data analysis and natural language processing
- Generative Adversarial Networks (GANs) for generating new content, such as images and text
- Transfer Learning for leveraging pre-trained models to solve similar tasks
- Autoencoders for dimensionality reduction and anomaly detection
Real-World Use Cases
Advanced deep learning techniques have been successfully applied to various real-world use cases, demonstrating their immense potential. Here are a few examples:
Medical Imaging Analysis
Deep learning models have been used to analyze medical images, such as X-rays and MRI scans, for accurate diagnosis of diseases and conditions. By leveraging CNNs, these models can detect abnormalities and assist healthcare professionals in making more informed decisions.
Natural Language Processing
RNNs have revolutionized natural language processing tasks, such as machine translation, sentiment analysis, and chatbots. These models can understand context, semantics, and even generate human-like text, greatly advancing the field of language understanding and generation.
Financial Market Prediction
Deep learning models combined with financial data can provide valuable insights for predicting stock market movements and making informed investment decisions. By training models on historical market data, these techniques can uncover hidden patterns and trends.
Autonomous Vehicles
Deep learning plays a vital role in the development of self-driving cars. By analyzing sensor data, such as camera images and LiDAR scans, deep learning models can detect objects, understand road scenes, and make real-time decisions, enabling safer and more efficient autonomous driving systems.
Advanced deep learning with Tensorflow and Keras has revolutionized the field of artificial intelligence and machine learning. The combination of Tensorflow's power and flexibility with Keras' simplicity has enabled developers to push the boundaries of what's possible. By applying advanced techniques and leveraging real-world use cases, deep learning models can tackle complex problems and provide valuable solutions across various industries. So, whether you're a beginner or an experienced practitioner, now is the time to dive into the world of advanced deep learning!
4.4 out of 5
Language | : | English |
File size | : | 28714 KB |
Text-to-Speech | : | Enabled |
Screen Reader | : | Supported |
Enhanced typesetting | : | Enabled |
Print length | : | 512 pages |
Updated and revised second edition of the bestselling guide to advanced deep learning with TensorFlow 2 and Keras
Key Features
- Explore the most advanced deep learning techniques that drive modern AI results
- New coverage of unsupervised deep learning using mutual information, object detection, and semantic segmentation
- Completely updated for TensorFlow 2.x
Book Description
Advanced Deep Learning with TensorFlow 2 and Keras, Second Edition is a completely updated edition of the bestselling guide to the advanced deep learning techniques available today. Revised for TensorFlow 2.x, this edition introduces you to the practical side of deep learning with new chapters on unsupervised learning using mutual information, object detection (SSD),and semantic segmentation (FCN and PSPNet),further allowing you to create your own cutting-edge AI projects.
Using Keras as an open-source deep learning library, the book features hands-on projects that show you how to create more effective AI with the most up-to-date techniques.
Starting with an overview of multi-layer perceptrons (MLPs),convolutional neural networks (CNNs),and recurrent neural networks (RNNs),the book then introduces more cutting-edge techniques as you explore deep neural network architectures, including ResNet and DenseNet, and how to create autoencoders. You will then learn about GANs, and how they can unlock new levels of AI performance.
Next, you’ll discover how a variational autoencoder (VAE) is implemented, and how GANs and VAEs have the generative power to synthesize data that can be extremely convincing to humans. You'll also learn to implement DRL such as Deep Q-Learning and Policy Gradient Methods, which are critical to many modern results in AI.
What you will learn
- Use mutual information maximization techniques to perform unsupervised learning
- Use segmentation to identify the pixel-wise class of each object in an image
- Identify both the bounding box and class of objects in an image using object detection
- Learn the building blocks for advanced techniques - MLPss, CNN, and RNNs
- Understand deep neural networks - including ResNet and DenseNet
- Understand and build autoregressive models – autoencoders, VAEs, and GANs
- Discover and implement deep reinforcement learning methods
Who this book is for
This is not an introductory book, so fluency with Python is required. The reader should also be familiar with some machine learning approaches, and practical experience with DL will also be helpful. Knowledge of Keras or TensorFlow 2.0 is not required but is recommended.
Table of Contents
- Introducing Advanced Deep Learning with Keras
- Deep Neural Networks
- Autoencoders
- Generative Adversarial Networks (GANs)
- Improved GANs
- Disentangled Representation GANs
- Cross-Domain GANs
- Variational Autoencoders (VAEs)
- Deep Reinforcement Learning
- Policy Gradient Methods
- Object Detection
- Semantic Segmentation
- Unsupervised Learning Using Mutual Information
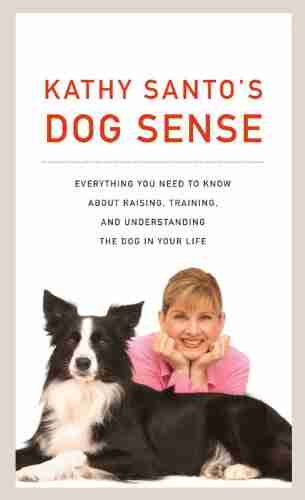

Kathy Santo Dog Sense Kathy Santo - Unlocking the secrets...
Are you a dog lover who...
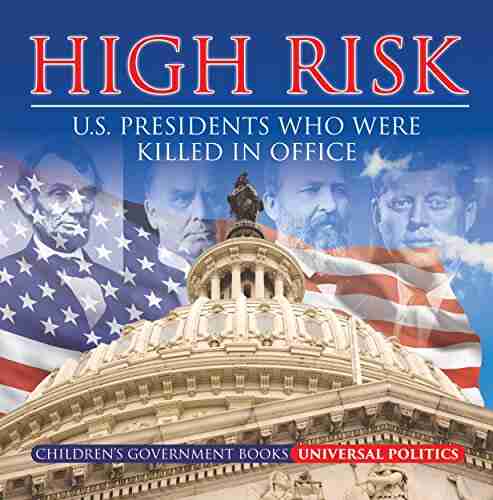

10 Presidents Who Were Killed In Office - Shocking Truth...
Throughout history, the role of a president...
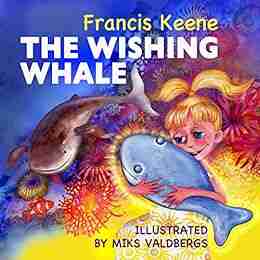

Unveiling a World of Magic: Beautifully Illustrated...
Bedtime stories have always held a...
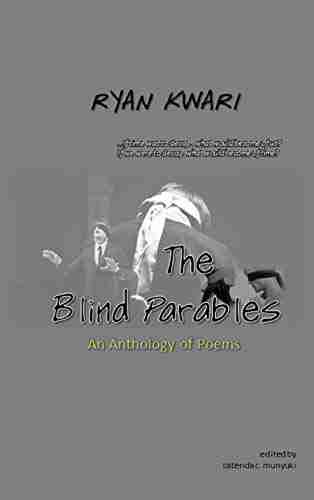

The Blind Parables: An Anthology Of Poems
For centuries, poetry has...
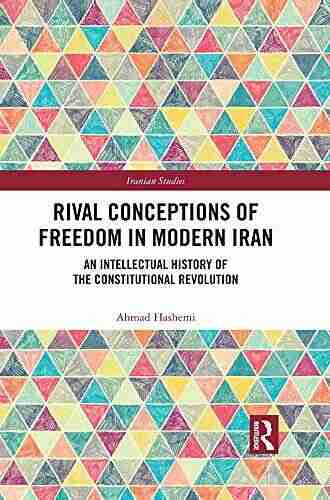

Rival Conceptions Of Freedom In Modern Iran
The Struggle for Freedom in...
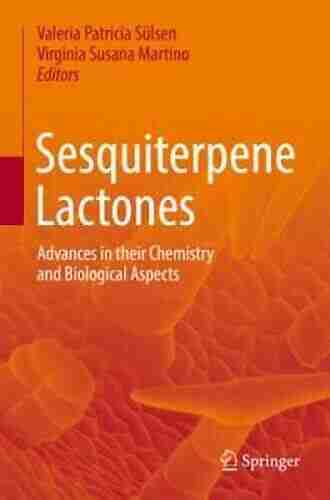

Advances In Their Chemistry And Biological Aspects
In recent years,...
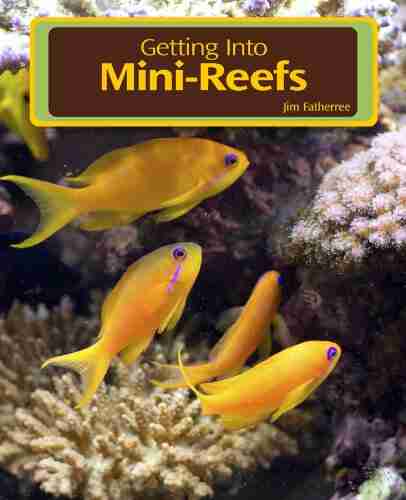

Getting Into Mini Reefs For The Marine Aquarium
Are you interested in enhancing the...
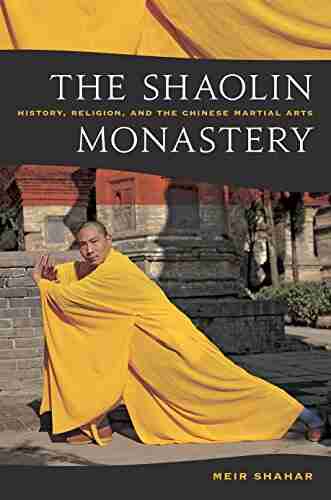

Exploring the Intriguing Connection Between History,...
When one thinks of Chinese martial...
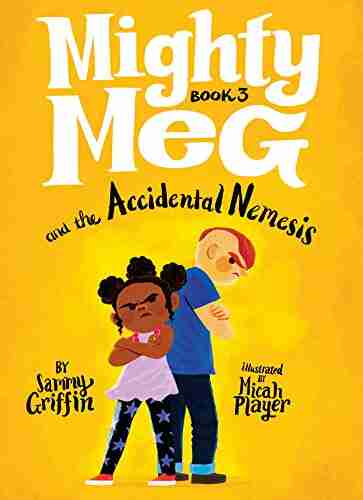

Mighty Meg And The Accidental Nemesis: Unleashing the...
In the world of superheroes, there are many...
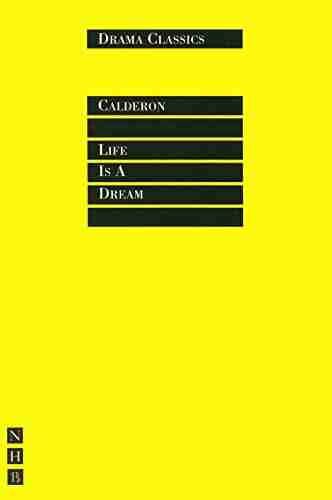

A Journey through the World of Nhb Drama Classics: Full...
Welcome to a fascinating exploration of Nhb...
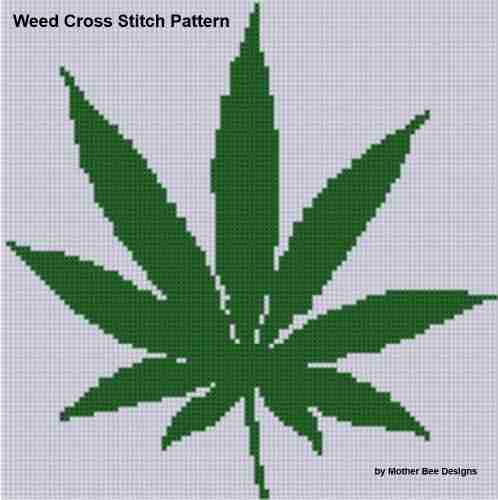

Weed Cross Stitch Pattern Rachel Worth - The Perfect...
Are you a stoner who loves a little...
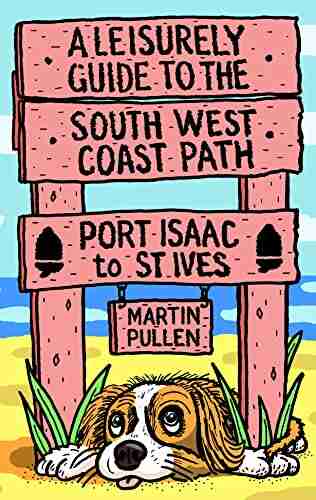

Discover the Breathtaking Beauty of the South West Coast...
Are you ready for an...
Light bulbAdvertise smarter! Our strategic ad space ensures maximum exposure. Reserve your spot today!
- Paul ReedFollow ·11.4k
- Kirk HayesFollow ·8.2k
- Harry HayesFollow ·7.5k
- John KeatsFollow ·8.1k
- Samuel Taylor ColeridgeFollow ·10.2k
- Brody PowellFollow ·17.5k
- Alfred RossFollow ·18.8k
- Andy HayesFollow ·5k